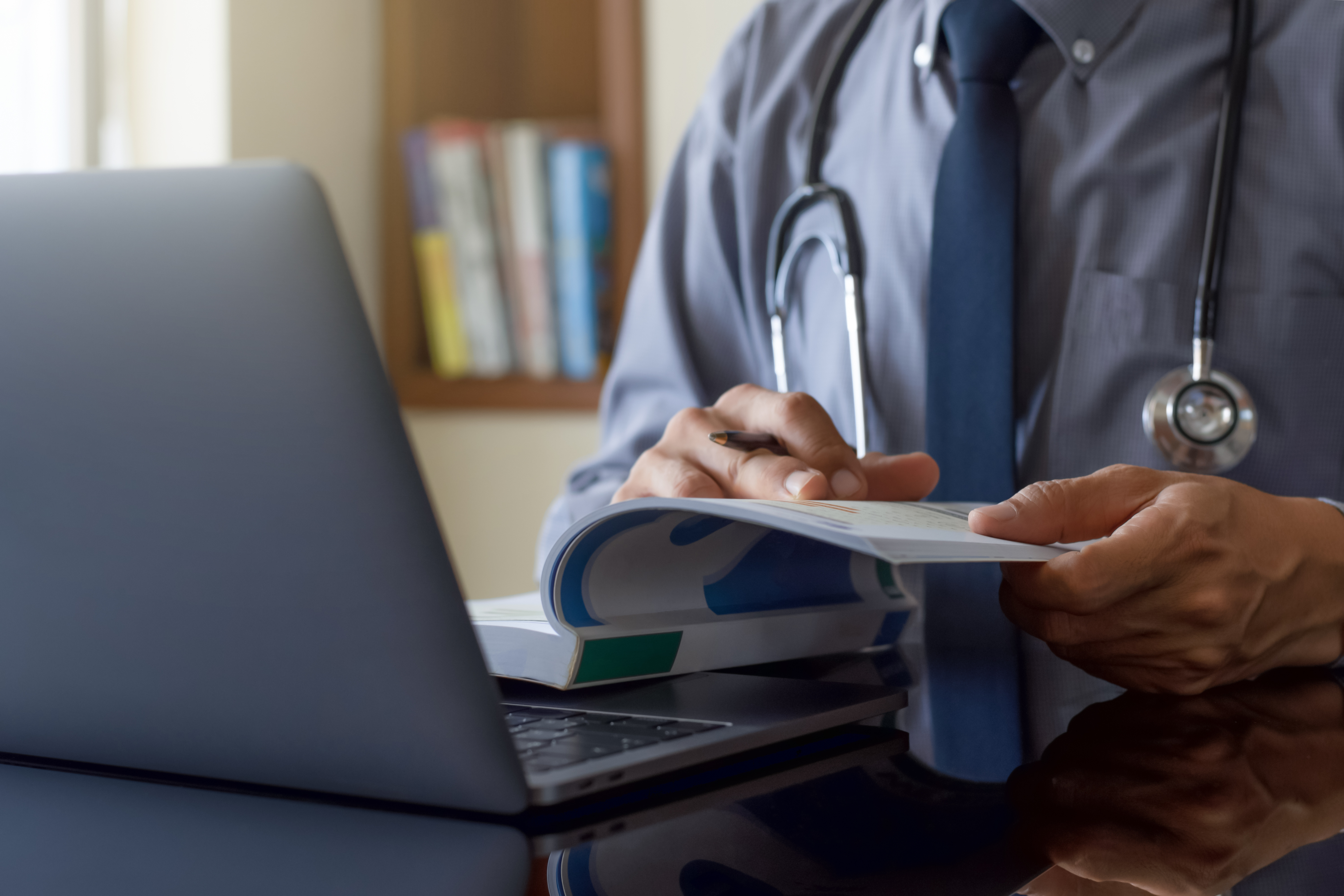
A new artificial intelligence (AI) framework determined that dermatology training materials—such as textbooks, lecture notes, and published articles—may not sufficiently include examples of dermatologic conditions in darker skin tones and could be contributing to disparities in dermatologic care for underserved populations, according to a study published by Tadesse et al in NPJ Digital Medicine. Manual assessments conducted to estimate the representation of darker skin tones in dermatologic training images can introduce human errors, and may not allow the assessments to cover a large quantity of educational materials. In the new study, researchers used the Skin Tone Analysis for Representation in Educational Materials (STAR-ED) framework to automate the assessment process. The STAR-ED framework works by parsing extract text, images, and table entities and employing machine learning to grade the skin tones within the images. After testing the STAR-ED framework on four popular medical textbooks, the researchers found that the framework was highly capable of detecting skin images (0.96 ± 0.02 average area under receiver operating characteristic [AUROC] and 0.90 ± 0.06 F1 score) and classifying skin tones (0.87 ± 0.01 average AUROC and 0.91 ± 0.00 F1 score). Further, the STAR-ED framework revealed that only 10.5% of all skin images were representative of darker skin tones. The researchers hope their new findings can reduce bias in dermatologic education, explain diagnostic delays and high mortality rates for skin diseases in patients with darker skin tones, and inspire the authors and editors of future dermatology training materials to improve inclusion of diverse skin tones.